Mastering Predictive Analytics in Sales: A Comprehensive Guide for Success
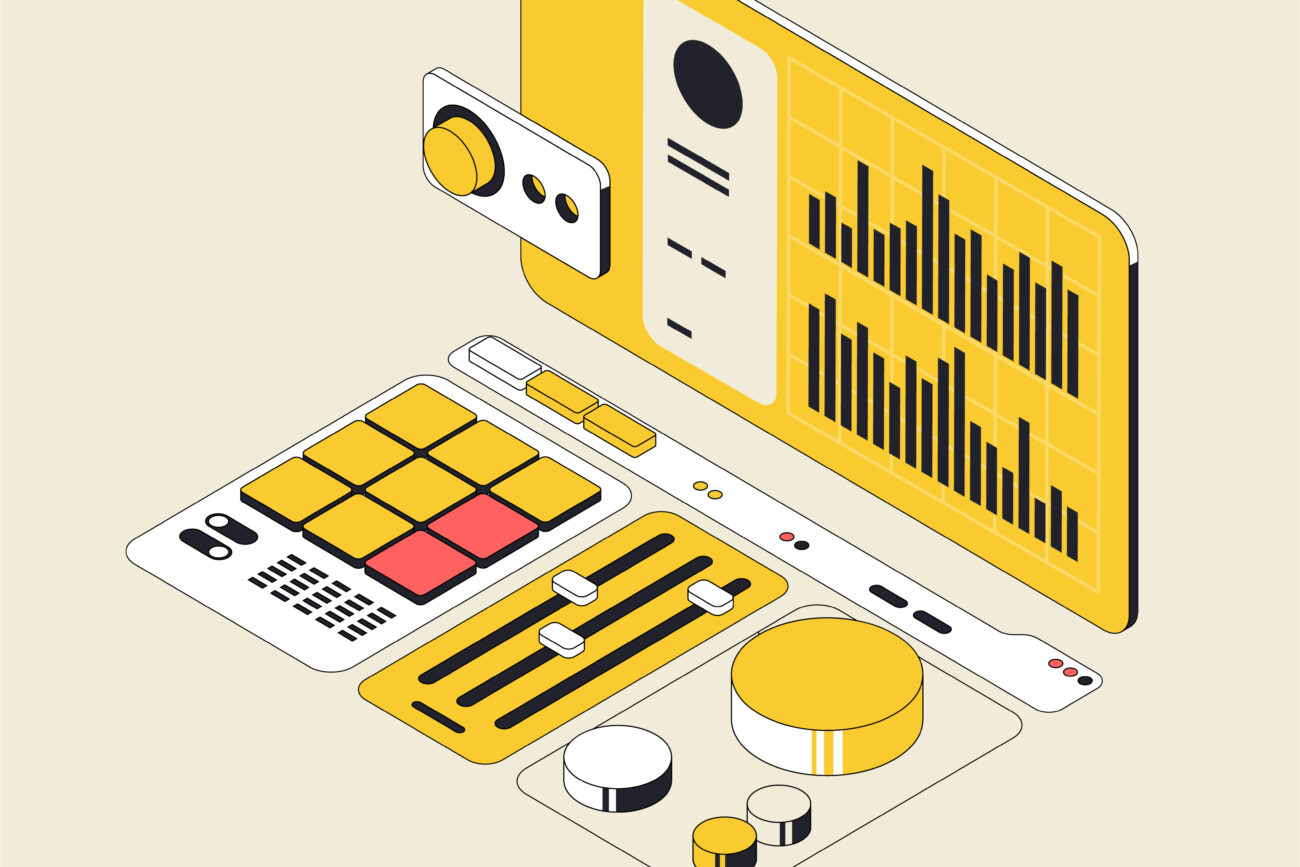
Effortlessly uncover thousands of high-value leads—no downloads, no hassle. Just enter your target keywords and let our tool do the work. find, contact, and close your ideal buyers
Grow Your Sales Pipeline With Human + AI, Drive sales growth with data-driven Custom Lead Generation Infrastructure, We will build for you a Lead generation machine with customizable workflows, API integrations, and intelligent automation for outreach, engagement, pipeline management & more.
In the dynamic landscape of sales, staying ahead of the competition requires more than just intuition and experience. Enter predictive analytics—a powerful tool revolutionizing sales strategies by leveraging historical data to forecast future outcomes. In this comprehensive guide, we’ll delve deep into the realm of predictive analytics in sales, exploring its definition, benefits, implementation steps, challenges, and real-world applications. Whether you’re a seasoned sales professional or just dipping your toes into the world of data-driven decision-making, this guide will equip you with the knowledge and tools needed to master predictive analytics and propel your sales efforts to new heights.
Section 1: Understanding Predictive Analytics in Sales
Definition of Predictive Analytics: Explaining the concept of predictive analytics and its significance in sales forecasting.
Benefits of Predictive Analytics: Highlighting the advantages of using predictive analytics in sales, including improved forecasting accuracy, lead scoring, and enhanced customer insights.
Real-World Examples: Illustrating the practical applications of predictive analytics in sales through case studies and success stories from leading organizations.
Section 2: Implementing Predictive Analytics in Sales
Step 1: Data Collection
Importance of Data Quality: Emphasizing the critical role of high-quality data in generating accurate predictions.
Data Sources: Identifying key data sources, including CRM systems, marketing automation platforms, product analytics tools, and third-party data providers.
Best Practices for Data Collection: Providing tips for collecting and organizing data effectively to ensure its relevance and accuracy.
Step 2: Choosing the Right Tools
Selection Criteria: Outlining the factors to consider when choosing predictive analytics tools, such as advanced modeling capabilities, ease of use, and integration with existing systems.
Recommended Tools: Highlighting industry-leading tools like HubSpot, Salesforce Einstein, and Breadcrumbs for their predictive modeling capabilities and ease of implementation.
Step 3: Defining Clear Objectives
Goal Setting: Stressing the importance of defining clear objectives and measurable goals before implementing predictive analytics.
Business Impact: Discussing how to align predictive analytics objectives with business goals and measure success through key performance indicators (KPIs).
Step 4: Continual Refinement
Adaptability: Emphasizing the need for regular updates and refinements to predictive models to accommodate changing market conditions and new data.
A/B Testing: Introducing the concept of A/B testing for evaluating the performance of different predictive models and refining strategies based on results.
Step 5: Training
User Training: Providing guidance on training sales teams to interpret and act on insights generated by predictive analytics tools.
Integration: Recommending tools that seamlessly integrate with existing tech stacks to minimize disruption and ensure smooth adoption by sales teams.
Section 3: Overcoming Challenges in Predictive Analytics
Challenge 1: Data Quality
Importance of Data Cleaning: Offering strategies for maintaining data quality through regular cleaning and validation processes.
Data Management Systems: Recommending robust data management systems to ensure accuracy and consistency in data collection and storage.
Challenge 2: Complexity
Simplification: Discussing the importance of user-friendly tools and training programs to simplify the implementation of predictive analytics for teams without data science expertise.
Expert Support: Suggesting options for accessing expert support or hiring data science professionals to manage complex predictive models.
Challenge 3: Ethical Considerations
Data Ethics Policy: Advocating for the development of clear data ethics policies to ensure responsible and ethical use of customer data.
Compliance: Highlighting the importance of compliance with data protection regulations and obtaining informed consent before collecting or using customer data.
In conclusion, mastering predictive analytics in sales is not just about adopting the latest technology—it’s about leveraging data-driven insights to make informed decisions and drive business success. By following the comprehensive guide outlined in this article, sales professionals can harness the power of predictive analytics to enhance forecasting accuracy, prioritize leads effectively, and optimize sales strategies for maximum impact. Embrace the future of sales with predictive analytics and embark on a journey towards unprecedented success.