Mastering Predictive Analytics for Sales Forecasting: A Comprehensive Guide
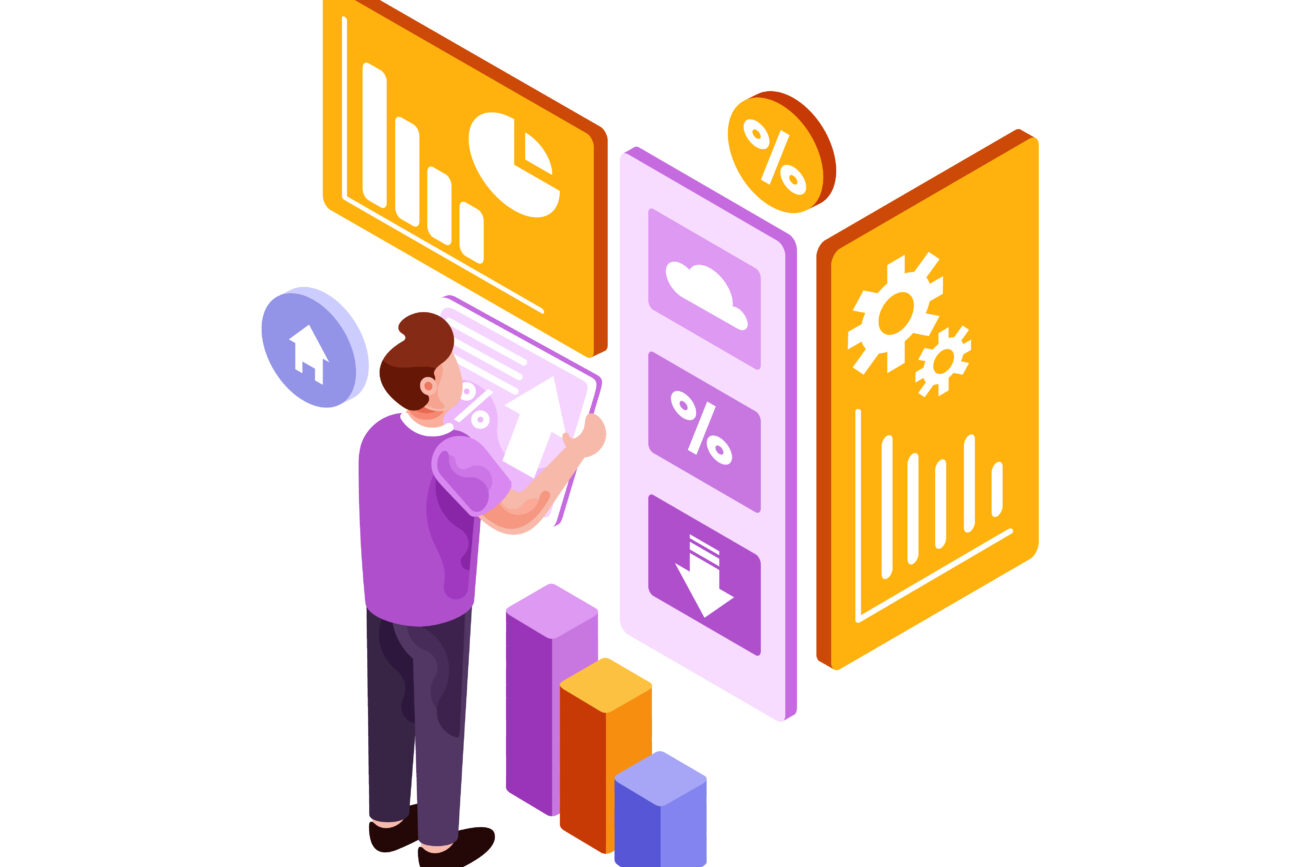
In today’s competitive business landscape, staying ahead of the curve requires more than just intuition. Predictive analytics has emerged as a game-changer for sales forecasting, empowering organizations to make data-driven decisions and achieve remarkable growth. In this comprehensive guide, we delve deep into the world of predictive analytics for sales forecasting, uncovering its benefits, practical applications, and best practices for implementation. Whether you’re a seasoned sales professional or a budding entrepreneur, this guide will equip you with the knowledge and tools to elevate your sales strategy to new heights.
Understanding Predictive Sales Forecasting
- Definition and Importance: Define predictive sales forecasting and elucidate its significance in revenue operations and strategic planning.
- Benefits: Explore the myriad benefits of predictive analytics in sales forecasting, from improved accuracy to enhanced decision-making.
- Traditional vs. Predictive Approach: Contrast traditional descriptive forecasting methods with proactive predictive analytics techniques, highlighting the shift towards a more dynamic and forward-looking approach.
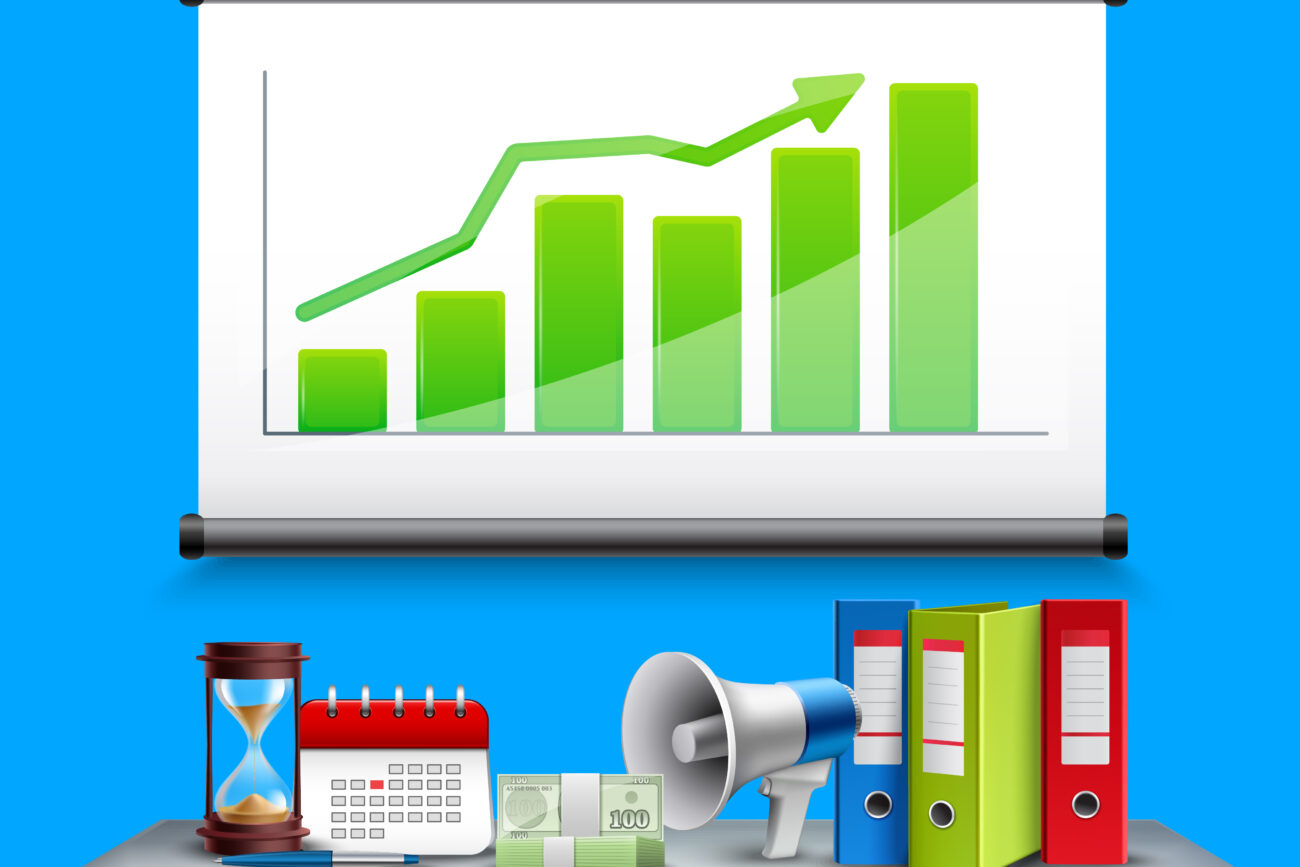
Implementing Predictive Analytics in Sales Forecasting
Data Sources and Integration: Discuss the various data sources crucial for predictive sales forecasting, including CRM data enhancement, email interaction insights, and AI-driven conversation tracking.
AI and Technology Integration: Explore the role of artificial intelligence and advanced analytics platforms in driving predictive modeling and dynamic forecasting adjustments.
Practical Steps: Provide actionable steps for implementing predictive analytics in sales forecasting, such as defining goals and metrics, choosing the right predictive model, and validating and refining the model.
Best Practices and Case Studies
Case Studies: Showcase real-world examples of organizations that have successfully leveraged predictive analytics for sales forecasting, illustrating the tangible benefits and ROI.
Expert Insights: Feature contributions from industry experts and thought leaders, offering valuable insights and practical advice for optimizing predictive sales forecasting strategies.
Emerging Trends: Highlight the latest trends and advancements in predictive analytics for sales forecasting, including AI-driven conversation tracking, dynamic pipeline change insights, and real-time business intelligence.
Maximizing the Impact of Predictive Sales Forecasting
Communication and Implementation: Discuss strategies for effectively communicating and implementing insights derived from predictive sales forecasting, ensuring alignment across departments and actionable decision-making.
Continuous Improvement: Emphasize the importance of continuous monitoring, evaluation, and refinement of predictive models to adapt to evolving market conditions and business dynamics.
Future Outlook: Offer a glimpse into the future of predictive analytics for sales forecasting, exploring emerging technologies and innovative approaches shaping the landscape.
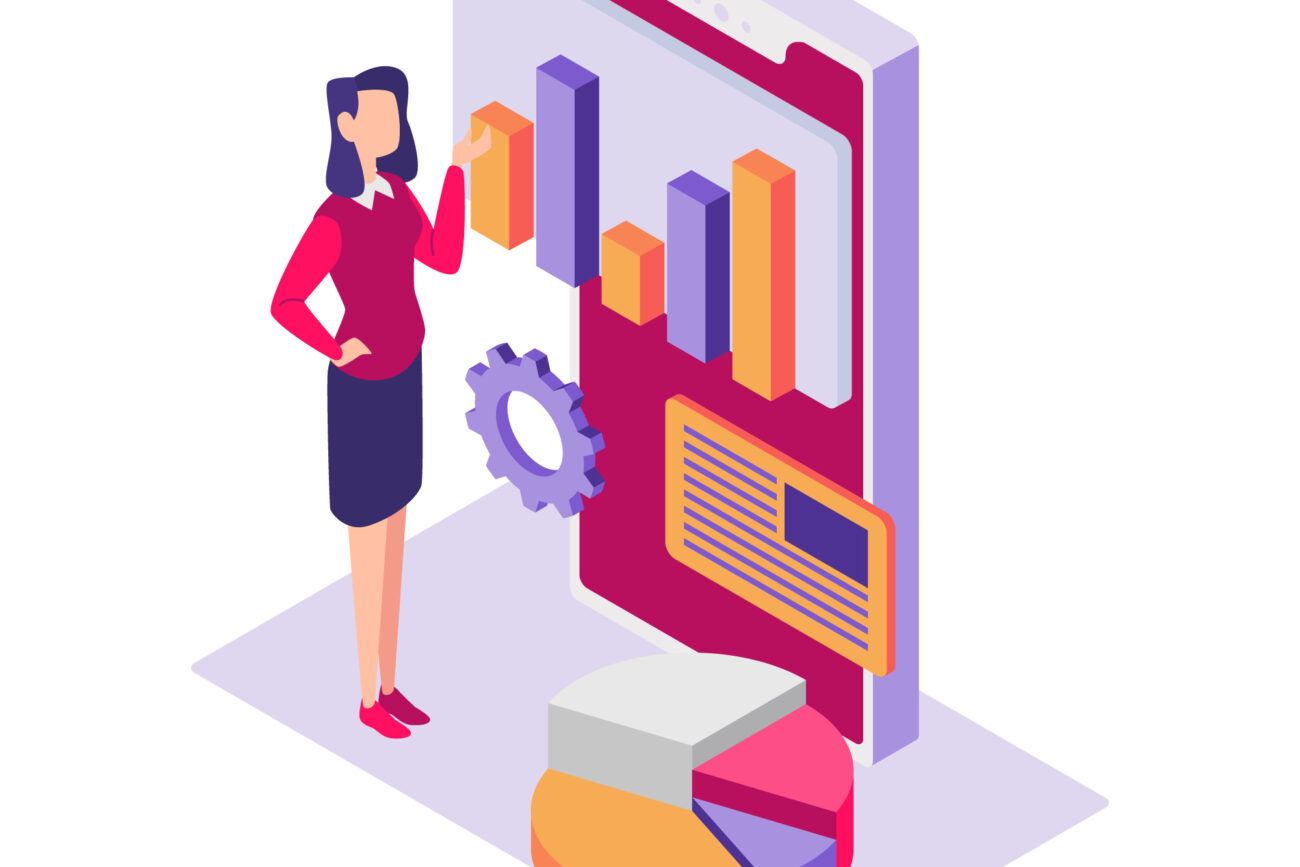
Overcoming Challenges and Pitfalls
– Data Quality and Accessibility: Address the challenges associated with data quality and accessibility, emphasizing the importance of data governance and integration efforts to ensure reliable and comprehensive datasets.
– Change Management: Explore the organizational challenges and resistance to change that may arise during the implementation of predictive analytics for sales forecasting, offering strategies for overcoming resistance and fostering a culture of data-driven decision-making.
– Model Interpretability: Discuss the importance of model interpretability and transparency in gaining stakeholder buy-in and building trust in predictive sales forecasting models, advocating for the use of explainable AI techniques and clear communication of results.
Scaling Predictive Sales Forecasting Efforts
– Scalability Considerations: Examine scalability considerations for predictive sales forecasting efforts, including infrastructure requirements, resource allocation, and scalability of predictive models to accommodate growing datasets and business needs.
– Integration with Business Processes: Highlight the importance of integrating predictive sales forecasting efforts with existing business processes and workflows, ensuring seamless alignment with sales operations, marketing initiatives, and strategic planning activities.
– Training and Development: Discuss the need for ongoing training and development programs to empower sales teams and stakeholders with the skills and knowledge needed to leverage predictive analytics effectively, fostering a culture of continuous learning and improvement.
Measuring Success and ROI
– Key Performance Indicators (KPIs): Identify key performance indicators (KPIs) for evaluating the success and ROI of predictive sales forecasting initiatives, including metrics such as forecast accuracy, revenue growth, lead conversion rates, and customer satisfaction scores.
– ROI Calculation: Outline methods for calculating the return on investment (ROI) of predictive sales forecasting efforts, taking into account factors such as cost savings, revenue generation, and efficiency improvements.
– Continuous Monitoring and Optimization: Stress the importance of continuous monitoring and optimization of predictive models to maximize ROI and ensure ongoing alignment with business objectives, emphasizing the value of iterative improvements and data-driven decision-making.
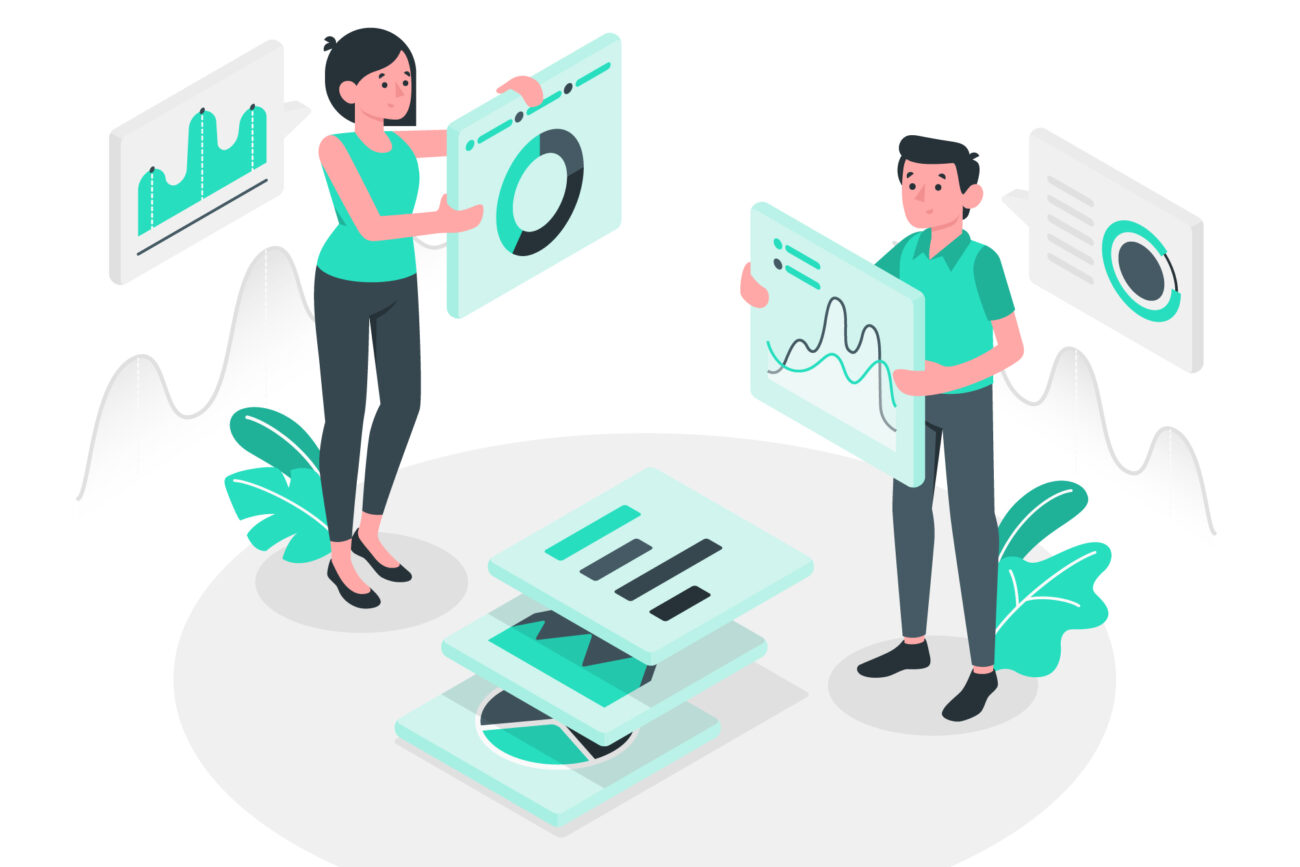
Future Directions and Emerging Trends
– AI and Machine Learning Advancements: Explore the latest advancements in artificial intelligence (AI) and machine learning (ML) technologies, such as deep learning algorithms, natural language processing (NLP), and predictive analytics platforms, and their potential impact on the future of sales forecasting.
– Predictive Analytics in Sales Enablement: Discuss the role of predictive analytics in sales enablement strategies, including personalized sales content recommendations, lead scoring automation, and predictive lead nurturing, and their implications for sales performance and productivity.
– Ethical Considerations: Address ethical considerations and implications associated with the use of predictive analytics in sales forecasting, including privacy concerns, bias mitigation, and responsible data stewardship practices, advocating for ethical AI principles and transparent decision-making processes.
In conclusion, mastering predictive analytics for sales forecasting requires a holistic approach that encompasses data-driven decision-making, technological innovation, organizational alignment, and continuous improvement. By overcoming challenges, scaling efforts, measuring success, and embracing emerging trends, businesses can unlock the full potential of predictive sales forecasting to drive growth, enhance competitiveness, and achieve sustainable success in today’s dynamic marketplace.